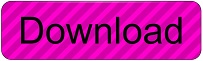
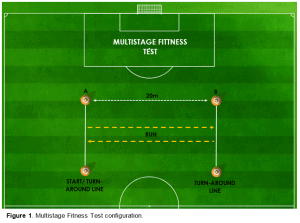
“There’s very good smart people on all sides of this debate,” says Ullman. Others (including himself and researchers such as Mitchell) are much more cautious. Some attribute the algorithms’ achievements to glimmers of reasoning, or understanding, he says. Other AI systems might beat the LLMs at any one task, but they have to be trained on data relevant to a specific problem, and cannot generalize from one task to another.ĬhatGPT is a black box: how AI research can break it openīroadly speaking, two camps of researchers have opposing views about what is going on under the hood of LLMs, says Tomer Ullman, a cognitive scientist at Harvard University in Cambridge, Massachusetts. What’s striking is the breadth of capabilities that emerges from this autocomplete-like algorithm trained on vast stores of human language. For chatbots built on LLMs, there is an extra element: human trainers have provided extensive feedback to tune how the bots respond. They work simply by generating plausible next words when given an input text, based on the statistical correlations between words in billions of online sentences they are trained on. In the past two to three years, LLMs have blown previous AI systems out of the water in terms of their ability across multiple tasks. “People in the field of AI are struggling with how to assess these systems,” says Melanie Mitchell, a computer scientist at the Santa Fe Institute in New Mexico whose team created the logic puzzles (see ‘An abstract-thinking test that defeats machines’). Tested another way, they seem less impressive, exhibiting glaring blind spots and an inability to reason about abstract concepts. Tested in one way, they breeze through what once were considered landmark feats of machine intelligence. The team behind the logic puzzles aims to provide a better benchmark for testing the capabilities of AI systems - and to help address a conundrum about large language models (LLMs) such as GPT-4.
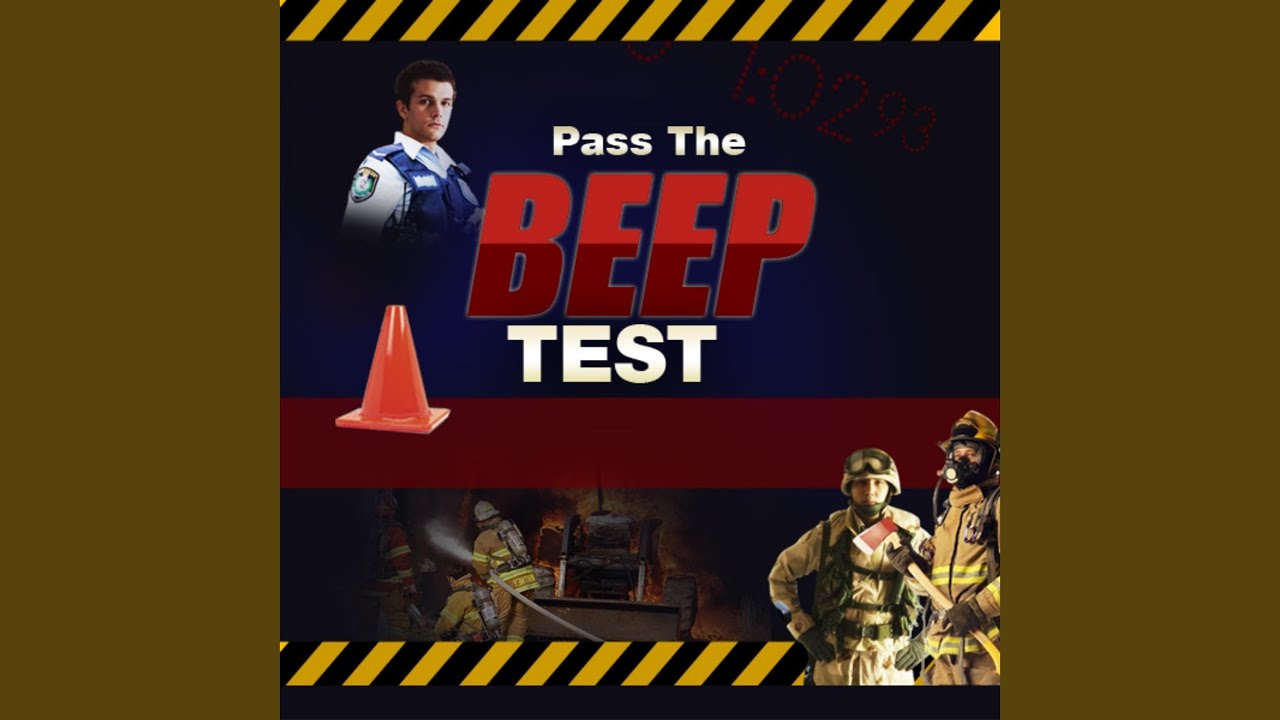
But GPT-4, the most advanced version of the AI system behind the chatbot ChatGPT and the search engine Bing, gets barely one-third of the puzzles right in one category of patterns and as little as 3% correct in another, according to a report by researchers this May 1. In a test consisting of a series of brightly coloured blocks arranged on a screen, most people can spot the connecting patterns. What can’t they do? Solve simple visual logic puzzles. The world’s best artificial intelligence (AI) systems can pass tough exams, write convincingly human essays and chat so fluently that many find their output indistinguishable from people’s.
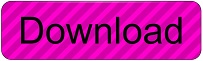